The article explores TCDModelSamplingDiscrete, a method used to select important data from larger sets, simplifying complex information for better analysis. It highlights its significance in various fields like healthcare, business, and education. The article explains how the method works, discusses common sampling techniques, and addresses challenges such as bias and representativeness. It encourages readers to learn more through books, online courses, and community engagement. The FAQs section answers common questions about it, emphasizing its importance in decision-making and data analysis. Overall, the article aims to provide a clear understanding of this valuable tool in data science.
What is TCDModelSamplingDiscrete?
TCDModelSamplingDiscrete is a special way to look at data. It helps us take a big group of information and pick out specific pieces that we need. Imagine you have a huge bag of candies, and you want to select just the red ones. TCDModelSamplingDiscrete does something similar but with numbers and data. It makes it easier for us to focus on what is important without getting lost in too much information.
When we use it, we can gather meaningful insights. This method is commonly used in different fields, like science, business, and education. By picking the right pieces of data, we can understand trends, make predictions, and even solve problems. This technique is like having a flashlight in a dark room—it helps us see what we need!
How Does TCDModelSamplingDiscrete Work?
Understanding how it works is quite simple. First, we collect data from different sources. This data can be numbers, survey answers, or even observations. After we have our data, we choose the important parts. This selection process is where TCDModelSamplingDiscrete shines.
Next, we analyze the selected data. This means we look closely at it to find patterns and learn from it. For example, if we selected data about students’ test scores, we could see which subjects they did well in and which ones they struggled with. By using it, we can turn raw data into useful information.
The Importance of TCDModelSamplingDiscrete in Data Analysis
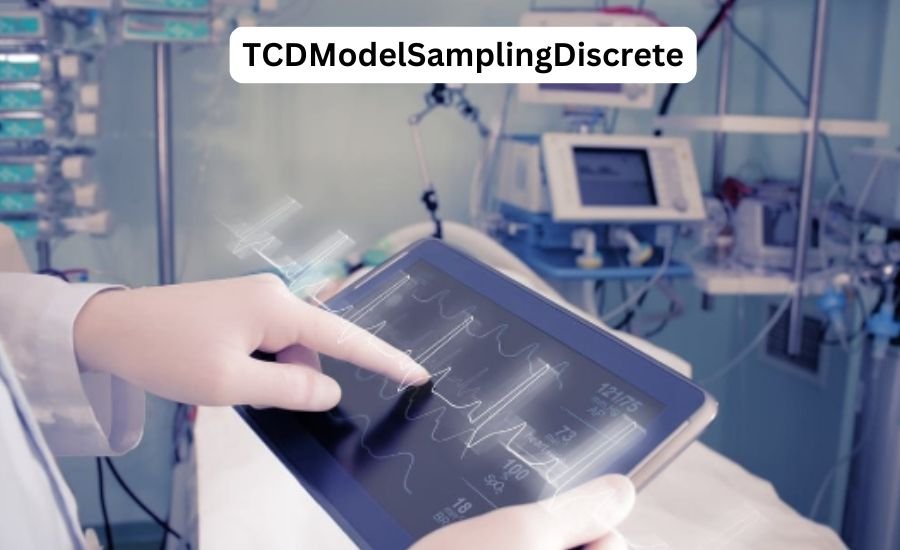
TCDModelSamplingDiscrete plays a crucial role in data analysis. Without it, we might drown in too much information and not know what to focus on. This method helps us filter out the noise and find the most relevant data. It’s like cleaning up a messy room so we can find our favorite toys!
In many businesses, it helps in making important decisions. For instance, a company may want to know which products are selling well. By using this sampling method, they can gather data on sales and discover the top sellers. This information helps them plan better for the future and keep their customers happy.
Different Types of Sampling in TCDModelSamplingDiscrete
- Random Sampling: In random sampling, each piece of data has an equal chance of being chosen. This method helps ensure that the sample is fair and representative, giving a true picture of the larger group.
- Systematic Sampling: Systematic sampling involves selecting every nth item from a list. For example, if we have a list of students, we might choose every 5th student. This method saves time while still providing useful results.
- Stratified Sampling: Stratified sampling divides the population into different groups, or strata, based on specific characteristics. Then, samples are taken from each stratum. This ensures that all groups are represented in the sample.
- Cluster Sampling: In cluster sampling, the population is divided into clusters, usually based on location or similar traits. Then, entire clusters are randomly selected for sampling. This method is useful when it’s hard to access the whole population.
- Convenience Sampling: Convenience sampling involves selecting data that is easy to access. While this method is quick and straightforward, it may not always be representative of the entire population, leading to biased results.
Understanding these different types of sampling methods in it can help us choose the best approach for our specific needs.
Benefits of Using TCDModelSamplingDiscrete
Versatility: TCDModelSamplingDiscrete can be applied in various fields such as business, healthcare, and education, improving results across different areas.
Efficiency: TCDModelSamplingDiscrete allows us to focus on a smaller, more manageable set of data rather than analyzing huge amounts. This makes our work quicker and easier.
Accuracy: By selecting specific data points, we reduce the chances of making mistakes, leading to more reliable conclusions.
Improved Understanding: Using this method enhances our comprehension of complex data, allowing us to identify trends and patterns more effectively.
Time-Saving: With a smaller dataset to analyze, we save time while still gaining valuable insights.
Common Mistakes in TCDModelSamplingDiscrete
Even though TCDModelSamplingDiscrete is useful, there are common mistakes people make. One mistake is not selecting a representative sample. If we only choose data that confirms what we believe, we might miss out on important information. This can lead to incorrect conclusions and poor decisions.
Another mistake is using too small a sample size. If our sample is too small, it may not reflect the larger group accurately. It’s important to find the right balance so that we can gain meaningful insights from our data. Learning from these mistakes can help us improve our use of this powerful method.
Tools and Software for TCDModelSamplingDiscrete
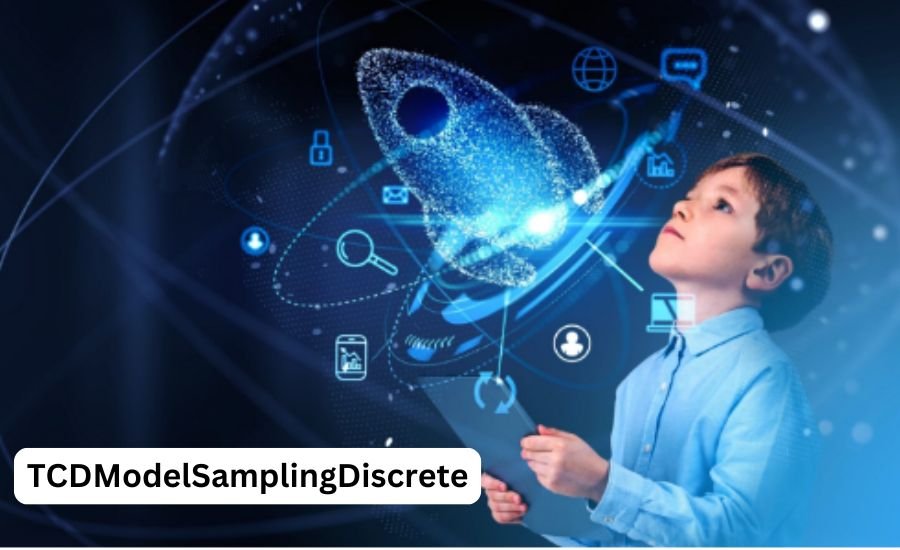
Many tools and software can help with it. These tools make it easier to collect and analyze data. Some popular software options include Excel, SPSS, and R. Each of these tools has features that can simplify the sampling process.
Using these tools, you can automate data collection and analysis. This saves time and reduces errors. Plus, they often come with helpful tutorials and guides. This makes it easier for anyone to start using TCDModelSamplingDiscrete effectively.
How TCDModelSamplingDiscrete Improves Decision Making
TCDModelSamplingDiscrete can greatly improve decision-making. When we have clear and relevant data, we can make better choices. For example, a restaurant owner might use this method to find out which dishes customers love the most. With this information, they can create a menu that keeps everyone happy.
In businesses, using it helps leaders understand market trends. This knowledge can lead to better marketing strategies and product development. By making informed decisions, organizations can achieve their goals more effectively.
Comparing TCDModelSamplingDiscrete to Other Sampling Methods
When we compare TCDModelSamplingDiscrete to other sampling methods, we see some clear differences. For instance, random sampling gives every data point an equal chance of being selected. This can lead to more balanced results. In contrast, convenience sampling might only select easy-to-reach data points. This could introduce bias.
Another method is stratified sampling, which divides data into groups before sampling. This can be helpful when specific characteristics are important. Understanding these differences can guide us in choosing the best method for our needs.
Don’t Skip: Pj-Bowen-Lancaster-California
Challenges of TCDModelSamplingDiscrete
Despite its benefits, TCDModelSamplingDiscrete comes with challenges. One challenge is ensuring that the sample is truly representative. If we fail to select the right data, our results may not reflect the larger group. This can lead to misleading conclusions.
Another challenge is the potential for bias. If we allow personal opinions to influence our sampling choices, we may overlook critical data. Being aware of these challenges can help us take steps to minimize their impact and improve our results.
Future of TCDModelSamplingDiscrete in Data Science
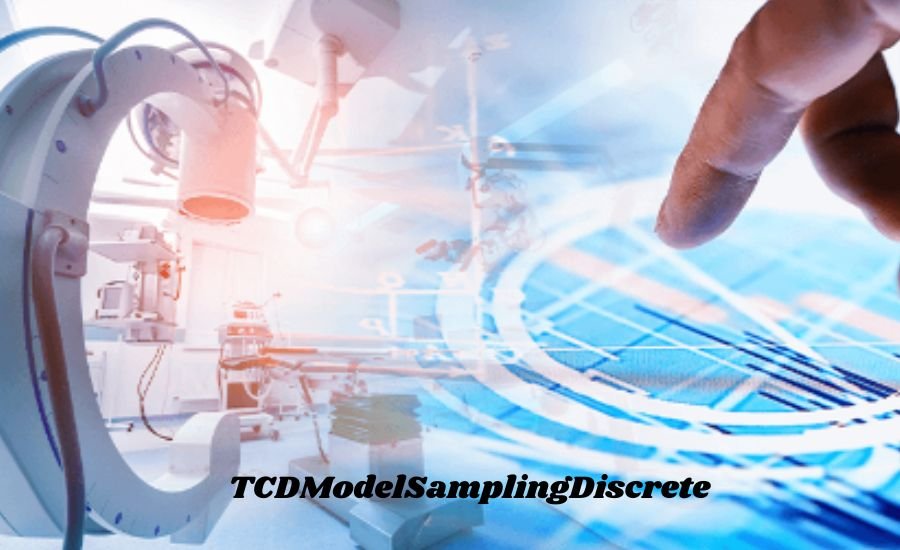
The future of TCDModelSamplingDiscrete in data science looks promising. As technology advances, we will have better tools to collect and analyze data. This will allow us to apply this method in new and exciting ways.
Additionally, as more people become interested in data science, TCDModelSamplingDiscrete will likely gain more attention. Educational programs will help teach the importance of this method. This will lead to more informed decision-making in various fields, making our world a better place.
Real-World Examples of TCDModelSamplingDiscrete
Real-world examples of TCDModelSamplingDiscrete are all around us. For instance, companies often conduct surveys to understand customer satisfaction. By sampling responses, they can learn what customers like or dislike about their products. This helps them make improvements and keep their customers happy.
In healthcare, researchers might study a disease’s impact by sampling data from patients. By analyzing this data, they can identify trends and develop better treatments. These examples show just how powerful TCDModelSamplingDiscrete can be in understanding the world around us.
Conclusion
In conclusion, TCDModelSamplingDiscrete is a vital tool for anyone working with data. It helps us focus on the most important information and make informed decisions. By understanding what TCDModelSamplingDiscrete is and how it works, we can use it effectively in many fields, from healthcare to education and business.
The benefits of TCDModelSamplingDiscrete are numerous, including increased efficiency and accuracy. However, it’s essential to be aware of common mistakes and challenges to maximize its potential. By implementing TCDModelSamplingDiscrete thoughtfully, we can improve our understanding of data and enhance decision-making.
As you continue your journey into the world of data analysis, remember the importance of learning and practicing. Whether through courses, reading, or engaging with communities, every step will help you become more proficient in using TCDModelSamplingDiscrete. So, embrace the journey and enjoy discovering the wonders of data!
Read Next: Tesoro-Silver-Sabre-Umax-Review-Amazon
FAQs
Q: What is TCDModelSamplingDiscrete?
A: TCDModelSamplingDiscrete is a method used to select specific pieces of data from a larger set. It helps simplify complex information, making it easier to analyze and understand.
Q: Why is TCDModelSamplingDiscrete important?
A: This method is important because it allows us to focus on the most relevant data, helping us make better decisions in fields like business, education, and healthcare.
Q: How does TCDModelSamplingDiscrete work?
A: It works by collecting data and then selecting specific parts of that data for analysis. This selection process helps highlight important trends and insights.
Q: What are some common sampling methods used in TCDModelSamplingDiscrete?
A: Common sampling methods include random sampling, where each item has an equal chance of being selected, and systematic sampling, where every nth item is chosen from a list.
Q: Can TCDModelSamplingDiscrete be used in healthcare?
A: Yes, TCDModelSamplingDiscrete is often used in healthcare to analyze patient data, understand disease patterns, and improve treatment methods.
Q: What challenges might arise when using TCDModelSamplingDiscrete?
A: Challenges include ensuring the sample is representative and avoiding bias in data selection. These factors can affect the accuracy of the results.
Q: How can I learn more about TCDModelSamplingDiscrete?
A: You can learn more by reading books and articles, taking online courses, or joining forums where data analysis is discussed. Engaging with the community can also enhance your understanding.