Understanding the r2 calculation using devsq and prediction multiple is essential for anyone analyzing data. R², or R-squared, tells us how well our predictions explain the data we observe. When we use devsq, we measure the squared differences between predicted and actual values, allowing us to assess the accuracy of our models effectively. This process is crucial in fields like business, science, and education.
At Urban Insightz, we delve deep into various data analysis methods, providing insights that empower our readers to make informed decisions. Our blog offers guidance on improving your understanding of R² and how it can enhance your predictive models. Whether you’re a beginner or an expert, our resources can help you grasp complex concepts easily, making data work for you.
What is r2 calculation using devsq and prediction multiple?
R-squared, often written as R², is a special number that helps us understand how well our predictions work. The R² calculation using devsq and prediction multiple tells us how much of the change in our data can be explained by our model. It can go from 0 to 1. If it is 1, it means our model is perfect and explains everything! If it is 0, our model does not help at all.
This calculation is very useful in many fields, like science and business. For example, if a scientist wants to know how different things affect plant growth, they can use R². By using devsq, we look at how far our guesses are from the real numbers. This helps us see if we need to change our predictions to make them better.
Why is R² Important in Statistics?
Understanding the importance of R² is key in statistics. It shows us if our predictions are good or not. When we use the r2 calculation using devsq and prediction multiple, we can decide if our model is worth using. A high R² value means our model does a good job at explaining the data. This can help researchers and businesses make better decisions.
When making decisions based on data, we want to be sure our model is reliable. If R² is low, it might be time to rethink our approach. By using this number, we can compare different models and choose the best one. This is why many analysts pay close attention to R² in their work.
How to Calculate R² Step by Step?
Calculating R² can seem tricky, but it is easier than it looks. First, we need to gather our data. We will need the actual values and the predicted values from our model. Once we have these, we can start the r2 calculation using devsq and prediction multiple.
Next, we will find the total variation in our data. This tells us how much the actual values change. Then, we will look at the explained variation, which is how much of that change our model can explain. After that, we can plug these numbers into the R² formula. Finally, we can see how well our model performed!
Understanding Deviation Squares (DevSq)
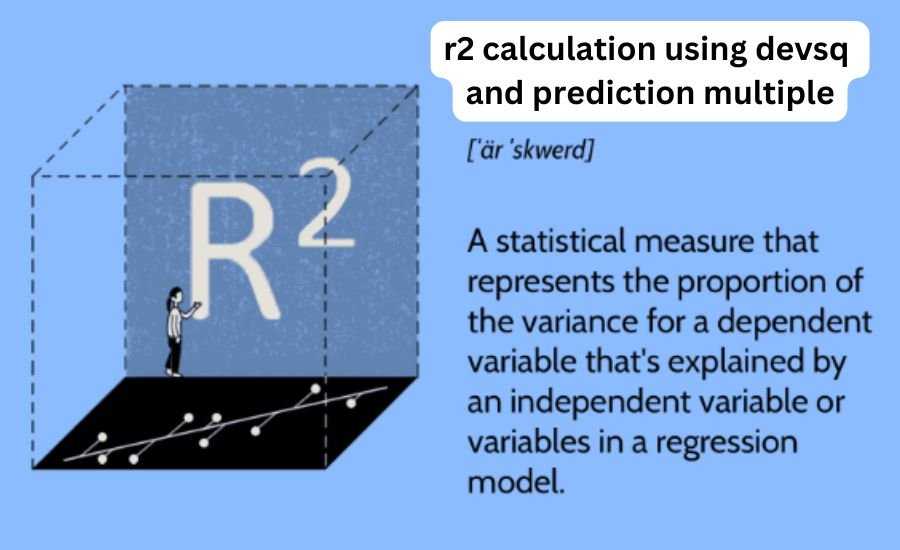
Deviation squares, often called devsq, are important for calculating R². They help us measure how much our predictions differ from the real values. When we talk about r2 calculation using devsq and prediction multiple, we often start by calculating the squares of these differences. This is how we get a clearer picture of how accurate our predictions are.
To find the deviation square, we take the difference between the actual value and the predicted value, then square that number. By squaring the differences, we make sure that all the numbers are positive. This way, we can focus on how far off our predictions are without worrying about positive or negative signs.
The Role of Prediction Multiple in R² Calculation
When we use multiple predictors, we can improve our predictions. The r2 calculation using devsq and prediction multiple allows us to see how well these different predictors work together. By adding more predictors, we can often get a better R² value, which means our model explains more of the data.
Each predictor helps us understand a different part of the data. For example, if we want to predict house prices, we might use predictors like size, location, and age of the house. Each of these factors can help us get closer to the actual price. This is why using multiple predictors is very helpful in our calculations.
Examples of R² Calculation Using DevSq and Prediction Multiple
Let’s look at an example to make this clearer. Suppose we have data on how many hours students study and their test scores. By using the r2 calculation using devsq and prediction multiple, we can predict test scores based on study hours.
First, we would gather data and calculate the predicted scores. Then, we would find the deviation squares for each student. Finally, we would calculate R² to see how well study hours explain test scores. This kind of analysis can show teachers how study habits affect student performance.
Common Mistakes in R² Calculation
When calculating R², it’s easy to make mistakes. One common error is not using the correct data. If we mix up actual and predicted values, our R² will be wrong. Also, forgetting to square the differences in devsq can lead to mistakes.
Another mistake is assuming a high R² always means a good model. Sometimes, a high R² value can be misleading, especially if our model is too complex. It is important to not only look at R² but also to understand the context of our data.
How to Improve Your R² Value?
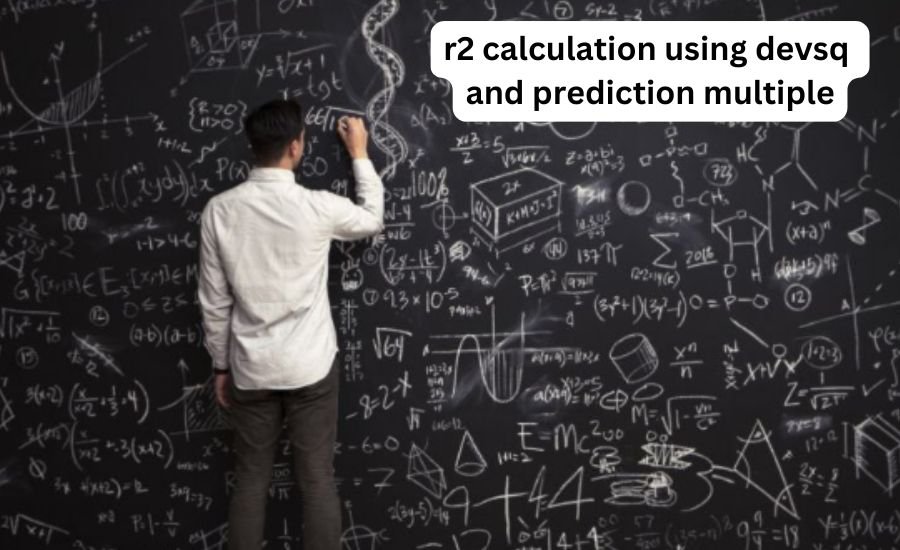
Improving R² is a goal for many analysts. One way to do this is by adding more relevant predictors to our model. The r2 calculation using devsq and prediction multiple often benefits from including new factors that may explain the data better.
We can also check if our data has any outliers. Outliers can greatly affect R², sometimes making it look better or worse than it really is. By cleaning our data and focusing on relevant information, we can often improve our R² and make our predictions more reliable.
Comparing Different Models Using R²
Comparing models using R² is a smart approach. We can use the r2 calculation using devsq and prediction multiple to see which model does a better job explaining the data. By running different models and calculating their R² values, we can pick the one that works best.
For example, if we are predicting sales, we might try different models with different predictors. By comparing R² values, we can see which model explains sales better. This helps businesses make informed decisions based on solid data.
Visualizing R²: Charts and Graphs
Visualizing R² can make understanding data easier. Using charts and graphs, we can show how well our predictions match the actual values. The r2 calculation using devsq and prediction multiple can be represented in different visual formats, like scatter plots.
In a scatter plot, we can plot actual values against predicted values. If the points are close to a straight line, it means our R² is high and our predictions are good. This visual representation helps everyone, even those who may not understand numbers well, to see how well the model is performing.
Real-World Applications of R² Calculation
R² has many real-world applications. It is widely used in areas like finance, health, and education. For example, in finance, analysts use R² to understand how different factors influence stock prices. The r2 calculation using devsq and prediction multiple helps them make better investment choices.
In healthcare, researchers use R² to study how different treatments affect patient recovery. By understanding the data better, they can improve healthcare outcomes. This shows how important R² is in various fields and its ability to inform real-life decisions.
Need To Know: Bogarete-bv-Men-Shoes-in-Chinese
R² Calculation in Excel: A Simple Guide
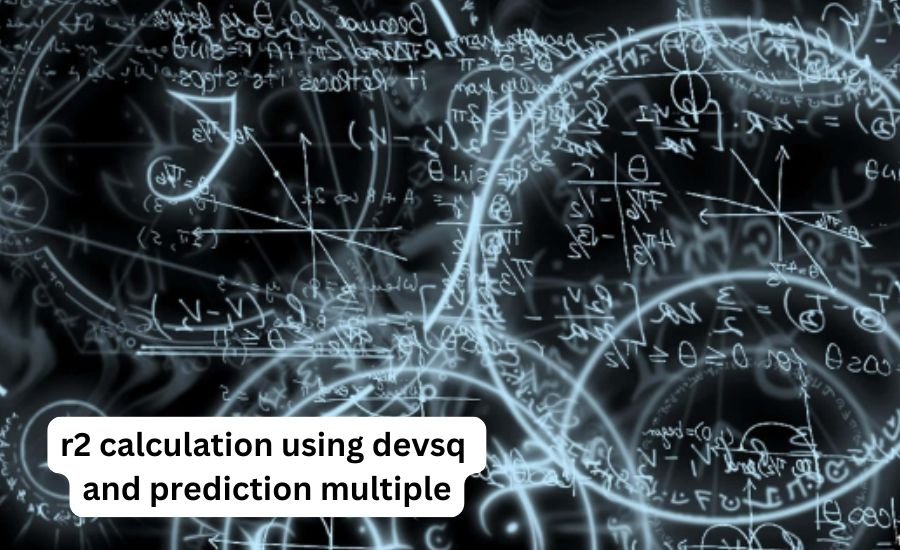
Using Excel to calculate R² can be simple and efficient. With just a few steps, we can perform the r2 calculation using devsq and prediction multiple right in our spreadsheets. First, we input our actual and predicted values into two columns.
Then, we can use the formula =RSQ(actual_range, predicted_range) to find R². Excel does the hard work for us! This makes it easy for anyone to analyze data without needing advanced math skills. Learning this technique can be very helpful for students and professionals alike.
Understanding Adjusted R² vs. Regular R²
Adjusted R² is another useful measure to understand. While regular R² shows how well a model explains the data, adjusted R² takes into account the number of predictors used. The r2 calculation using devsq and prediction multiple can sometimes give a misleading high value if too many predictors are included.
Adjusted R² helps us see if adding more predictors actually improves the model. It helps analysts avoid overfitting, where the model becomes too complex and doesn’t perform well on new data. This makes adjusted R² an important tool in data analysis.
How to Interpret R² Values
Interpreting R² values is crucial for understanding our results. A value close to 1 means our model does a great job explaining the data. However, if R² is low, it suggests we need to rethink our approach. The r2 calculation using devsq and prediction multiple helps us see where we stand.
It’s also important to consider the context of our data. A low R² might be acceptable in some fields, while in others, it could mean our model is not useful. Knowing how to interpret R² values helps us make informed decisions based on our analysis.
Conclusion
In conclusion, understanding the r2 calculation using devsq and prediction multiple is very important for anyone working with data. R² helps us see how well our predictions match the real world. By using devsq, we can measure the differences and find out if our models are doing a good job. This knowledge can help us make better decisions in many fields, like business, science, and education.
Moreover, using multiple predictors can improve our R² value and give us a clearer picture of the data. It’s not just about having a high R²; it’s about understanding what it means and how we can use it to inform our choices. With practice, anyone can learn to use R² effectively and make sense of their data. Keep exploring and learning, and soon you’ll be a pro at data analysis!
Read Next: Purple-and-Blue-Tie-Dye-Fugler
FAQs
Q: What does R² mean?
A: R², or R-squared, is a number that tells us how well our predictions explain the data. A high R² value means our model is good at making predictions.
Q: How is R² calculated?
A: R² is calculated by comparing the total variation in the data to the variation explained by our model. We often use devsq to find these values.
Q: What is devsq in R² calculation?
A: Devsq, or deviation squares, measures how far our predicted values are from the actual values. It helps us see the accuracy of our predictions.
Q: Why is R² important?
A: R² is important because it helps us decide if our model is useful. A high R² means we can trust our predictions.
Q: Can R² be too high?
A: Yes, if R² is too high, it might mean our model is too complex and could be overfitting. This means it works well on our data but not on new data.
Q: What is the difference between R² and adjusted R²?
A: R² measures how well a model explains the data, while adjusted R² accounts for the number of predictors used. Adjusted R² is often better for comparing models.